AI-Complete Question-Answering system based ML model bAbI: overiew (part-1)
The bAbI project is a machine-learning model by Facebook AI research
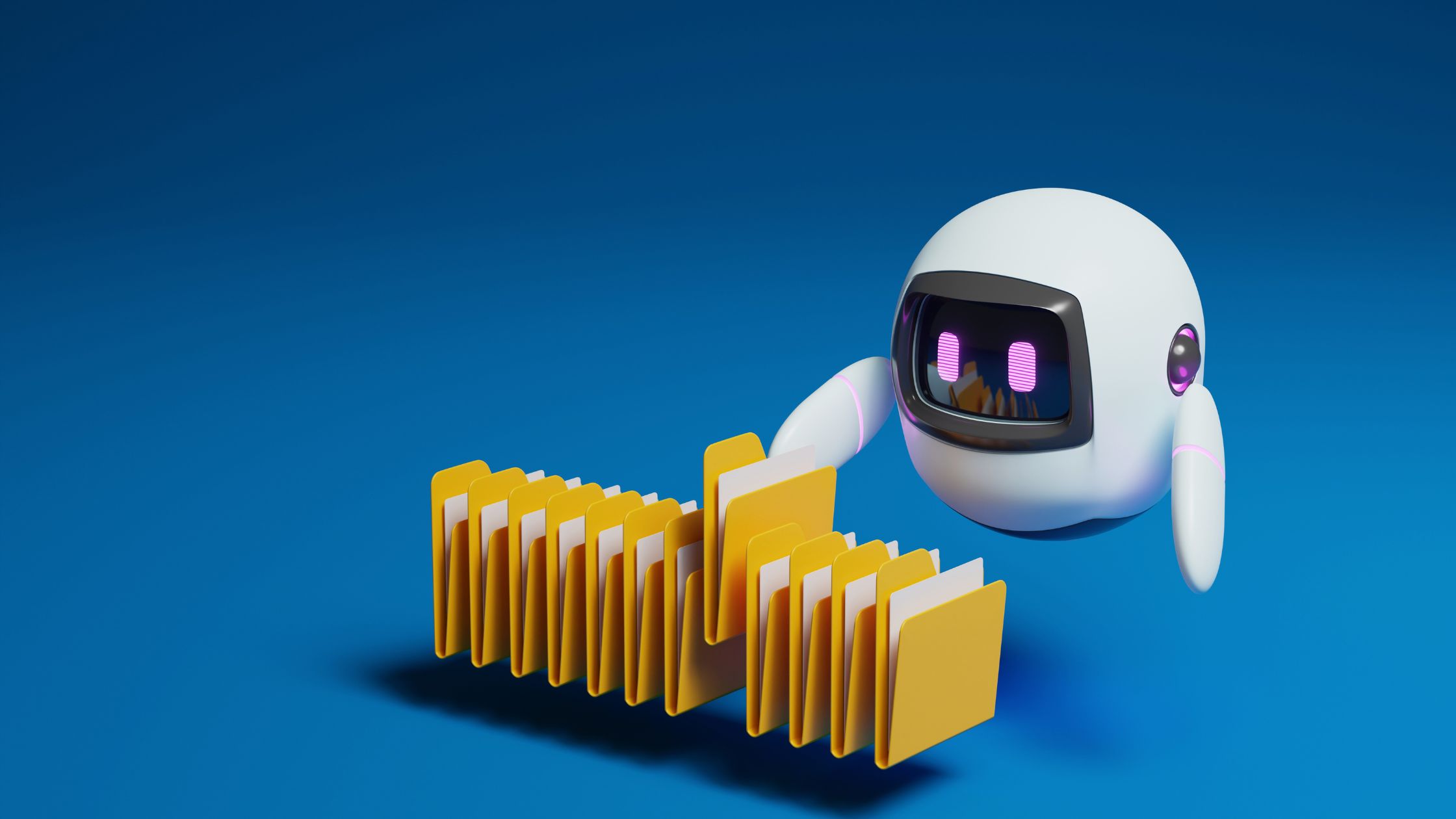
1. Deep learning models for Question-Answering
Some Keras-based models for QA on the babi dataset. Implemented in Keras 1.
- babi_memn2n.py: An end-to-end memory network implementation including:
- Bag of Word encoding.
- Multiple hops
- Adjacent embedding layers
- babi_rn.py: An relational network (RN) implementation for babi.
Source: https://github.com/jgpavez/qa-babi
2. End-to-End Memory Network for Goal-Oriented Conversational Agent
A Keras implementation with TensorFlow back end, tested on the Dialog bAbI dataset
This repository contains my unofficial Keras implementation of an End-to-End Memory Network [1] applied to Goal-Oriented dialog systems as described in Bordes et al [2]. The model can be trained on either of the five Dialog bAbI dataset [3]: set in the context of restaurant reservation, its tasks required manipulating sentences and symbols, so as to properly conduct conversations, issue API calls and use the information provided by the outputs of such calls.
It is implementaion some aspects of several goals in theoretical topic:
-
Learning END-TO-END goal-oriented dialog: Traditional dialog systems used in goal-oriented applications require a lot of domain-specific handcrafting, which hinders scaling up to new domains. Endto-end dialog systems, in which all components are trained from the dialogs themselves, escape this limitation. But the encouraging success recently obtained in chit-chat dialog may not carry over to goal-oriented settings.
-
End-To-End Memory Network: The architecture is a form of Memory Network but unlike the model in that work, it is trained end-to-end, and hence requires significantly less supervision during training, making it more generally applicable in realistic settings. It can also be seen as an extension of RNNsearch to the case where multiple computational steps (hops) are performed per output symbol. The flexibility of the model allows us to apply it to tasks as diverse as (synthetic) question answering and to language modeling. For the former our approach is competitive with Memory Networks, but with less supervision. For the latter, on the Text8 datasets our approach demonstrates comparable performance to RNNs and LSTMs. In both cases we show that the key concept of multiple computational hops yields improved results.
Source: https://github.com/candoz/memn2n-dialog-babi-keras
3. Goal Oriented Dialog System from voicy.AI
The objective of this project is to explore neural network architectures for question answering and goal oriented dialog systems. Will train on dialog bAbI tasks. Tensorflow is used for building the models. I have listed some of the influential/interesting papers below. If I have missed something, let me know.
- The dialog bAbI tasks
This section presents the set of 6 tasks for testing end-to-end dialog systems in the restaurant domain. Each task tests a unique aspect of dialog.
- Learning End-to-End Goal-Oriented Dialog
Source: https://github.com/voicy-ai/DialogStateTracking
4. Coupling Large Language Models with Logic Programming for Robust and General Reasoning from Text
While large language models (LLMs), such as GPT-3, appear to be robust and general, their reasoning ability is not at a level to compete with the best models trained for specific natural language reasoning problems. In this study, we observe that a large language model can serve as a highly effective few-shot semantic parser. It can convert natural language sentences into a logical form that serves as input for answer set programs, a logic-based declarative knowledge representation formalism. The combination results in a robust and general system that can handle multiple question-answering tasks without requiring retraining for each new task. It only needs a few examples to guide the LLM’s adaptation to a specific task, along with reusable ASP knowledge modules that can be applied to multiple tasks. We demonstrate that this method achieves state-of-the-art performance on several NLP benchmarks, including bAbI, StepGame, CLUTRR, and gSCAN. Additionally, it successfully tackles robot planning tasks that an LLM alone fails to solve.
Source: https://github.com/azreasoners/LLM-ASP